Mistakes to Avoid in Data Science Implementation for Startups
Posted By - Pony
Posted On - February 7, 2024
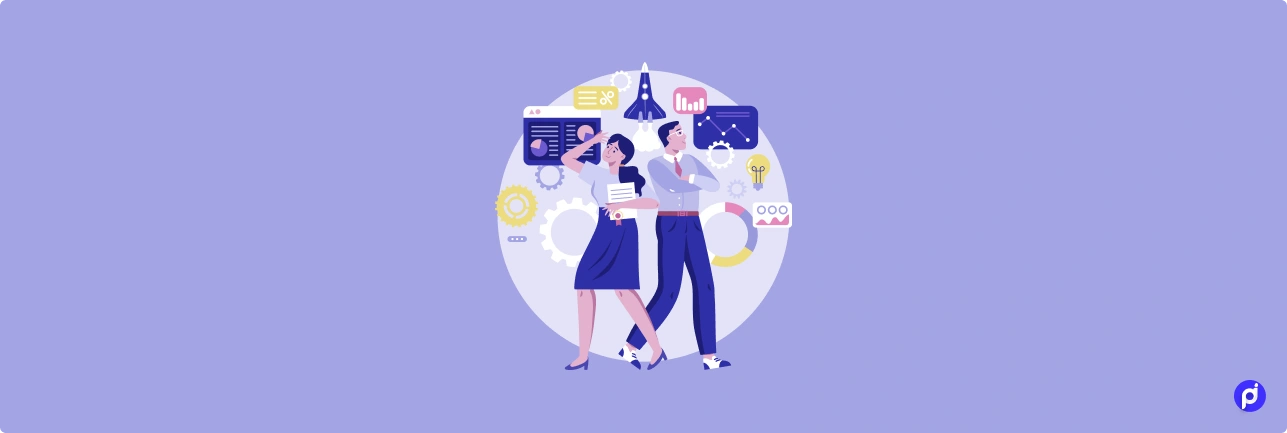
Startups are increasingly relying on data science services to gain a competitive edge and make informed decisions. However, the implementation of data science solutions is not without its challenges. Many startups make critical mistakes that can hinder the success of their data analytics initiatives. In this blog, we’ll explore some common pitfalls and offer insights on how startups can navigate the intricate landscape of data science implementation.
Important Mistakes to Avoid in Data Science Implementation:
1. Lack of Clear Objectives
One of the most common mistakes startups make in implementing data science solutions is the absence of clear objectives. Before diving into the world of data analytics, it’s crucial to define specific goals and outcomes. Whether it’s improving customer retention, optimizing operations, or enhancing product recommendations, a well-defined objective provides direction and focus for the data science team.
2. Neglecting Data Quality
Data is the lifeblood of any data science initiative. Startups often make the mistake of neglecting data quality, leading to inaccurate analyses and flawed insights. Investing time and resources in ensuring the cleanliness and accuracy of the data is essential. Data Science Services play a pivotal role in cleansing and transforming raw data into valuable insights, but they can only be effective with high-quality input.
3. Overlooking Scalability
Startups should not only focus on immediate data needs but also consider the scalability of their data science infrastructure. Growth of business leads to an increase in volume of data. Implementing scalable solutions ensures that the data infrastructure can handle increasing data loads without compromising performance. Enterprise Business Intelligence tools can be instrumental in creating scalable data architectures.
4. Ignoring Data Security
With the rising concerns about data breaches and privacy, startups cannot afford to ignore data security. It’s crucial to implement robust security measures to protect sensitive information. Encryption, access controls, and regular security audits should be integral parts of any data science implementation strategy.
5. Not Involving Stakeholders
Successful data science implementation requires collaboration and communication with key stakeholders. Many startups make the mistake of excluding non-technical teams from the process. Involving stakeholders from various departments ensures that the insights generated are aligned with the overall business strategy and meet the needs of different teams.
6. Rushing the Implementation Process
The excitement around leveraging data science can sometimes lead startups to rush the implementation process. It’s essential to take the time to plan, test, and iterate on the solution. A well-thought-out implementation strategy increases the chances of success and minimizes the risk of costly errors.
7. Underestimating Talent Requirements
Building a successful data science team requires skilled professionals with expertise in statistics, programming, and domain knowledge. Underestimating the talent requirements can result in a team that lacks the skills necessary for effective data analysis. Consider partnering with data science services to supplement in-house talent and access a broader skill set.
Finishing Off
While data science services offer immense potential for startups, avoiding these common mistakes is crucial for successful implementation. By setting clear objectives, prioritizing data quality, ensuring scalability, focusing on security, involving stakeholders, taking a methodical approach, and recognizing talent requirements, startups can harness the power of data science to drive informed decision-making and achieve sustainable growth.
The FAQ’s:
FAQ 1. Why is it essential for startups to define clear objectives before implementing data science solutions?
Startups often neglecting clear objectives can lead to directionless data analytics initiatives. Clearly defined goals, such as improving customer retention or optimizing operations, provide focus for the data science team and guide the entire implementation process.
FAQ 2. How can startups ensure the quality of data in their data science initiatives?
Neglecting data quality can result in inaccurate analyses. It’s crucial for startups to invest time and resources in ensuring the cleanliness and accuracy of their data. Data Science Services play a vital role in cleansing and transforming raw data into valuable insights, but high-quality input is essential.
FAQ 3. Why is scalability important in the context of data science infrastructure for startups?
Scalability ensures that a startup’s data science infrastructure can handle increasing data loads as the business grows without compromising performance. Implementing scalable solutions, possibly through Enterprise Business Intelligence tools, is essential for long-term success.
FAQ 4. What security measures should startups prioritize in their data science implementation strategy?
With rising concerns about data breaches and privacy, startups cannot afford to ignore data security. Encryption, access controls, and regular security audits should be integral parts of any data science implementation strategy to protect sensitive information.
FAQ 5. How crucial is stakeholder involvement in the success of data science implementation for startups?
Successful data science implementation requires collaboration with key stakeholders from various departments. Involving non-technical teams ensures that the insights generated align with the overall business strategy and meet the diverse needs of different teams within the startup.