Breaking Boundaries: How AI is Transforming the Landscape of Clinical Research
Posted By - Pony
Posted On - November 24, 2023
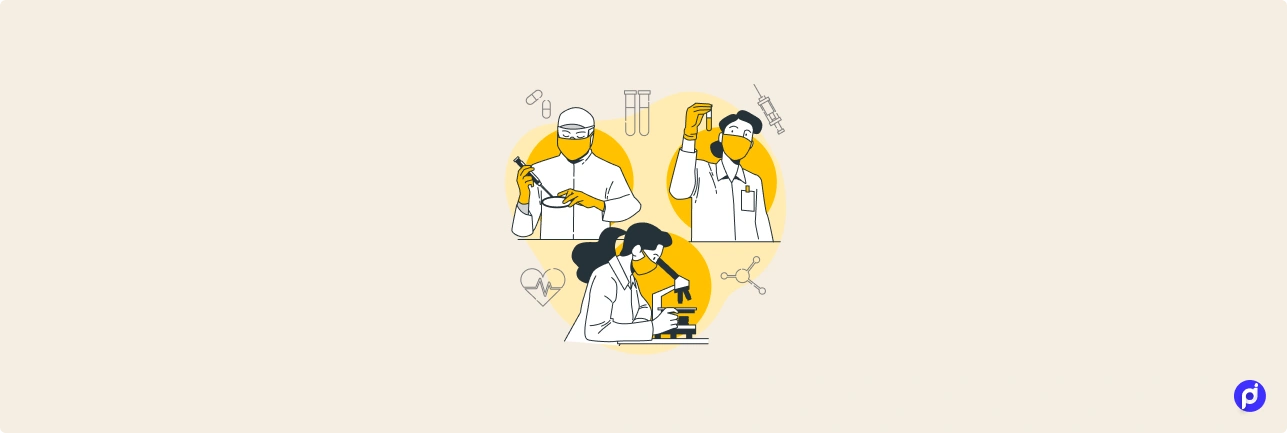
In the dynamic realm of healthcare, the convergence of artificial intelligence (AI) and clinical research has set the stage for a revolutionary transformation. As we navigate the intricate landscape of drug development and clinical trials, AI emerges as a powerful force, breaking traditional boundaries and ushering in an era of unprecedented possibilities.
The Evolution of Clinical Development
The roots of clinical development trace back to the mid-20th century with the introduction of randomized clinical trials (RCTs). These trials, while instrumental in establishing evidence-based drug development, have faced growing challenges in the contemporary era. The rigidity of RCTs, once a reliable defence against anecdotal evidence, is now viewed as a potential bottleneck, prolonging the approval process and escalating costs.
Enter AI, the catalyst for change.
From operational excellence to scientific advancements, AI is making its mark in clinical development. As the wave of innovation in pharmaceutical research surges forward, the pressure intensifies to align clinical development with the rapid strides made in AI, particularly in the realms of generative AI (gen AI) and foundational models.
According to recent research made by Roots Analysis,
“The global AI in clinical trials market is estimated to be worth US$ 8.50 Billion in 2035 growing at a compounded annual growth rate (CAGR) of 16% during the forecast period 2023-2035.”
The Challenge of Precision Medicine
Precision medicine, a paradigm shift in healthcare, demands a departure from the one-size-fits-all approach. RCTs are now confronted with the task of not only proving general treatment efficacy but also validating its effectiveness in specific patient segments. This shift, driven by the rise of precision medicine, adds complexity to trial enrollment, pushing researchers to design trials with greater precision.
Amid these changes, the demand for targeted and meaningful data has reached new heights. Patient populations are becoming increasingly segmented, making it challenging to enroll sufficient participants in trials. Regulatory standards, payer expectations, and competitive pressures have raised the bar for what constitutes a clinically meaningful treatment effect.
The Promise of AI in Clinical Development
As drug discovery accelerates with the aid of AI and gen AI, such as the remarkable AlphaFold by DeepMind predicting molecular structures, the spotlight turns to clinical development. The promise lies not only in operational efficiency but also in the strategic use of modern analytical tools and diverse data sources to design more precise and efficient trials with higher success rates.
Despite this potential, the adoption of AI in clinical development has primarily focused on operational enhancements and acceleration. Few established companies systematically deploy AI for trial design strategy. The shift from a focus on operational excellence to an informed trial design strategy is crucial for realizing the full benefits of AI in clinical research.
Expanding the Horizon with AI and Real-World Data (RWD)
Regulators have recognized the need for change, issuing guidance on the appropriate use of real-world data (RWD). The healthcare data ecosystem is flourishing, ensuring privacy-respecting technology facilitates the availability of patient-level data for research. Causal machine learning, blending biostatistics with machine learning and gen AI, is distinguishing correlation from causation.
In the present landscape, AI draws upon a wealth of RWD, including electronic health records, claims data, biobanks, omics panels, genomic studies, patient registries, and imaging. Patient data sharing has reached new heights, respecting privacy while enabling the training of ML models. Large language models, exemplified by BioGPT, transform unstructured physician notes into high-quality structured data, contributing to a comprehensive understanding of available evidence.
Unlocking the Potential: Use Cases Illustrating AI Impact
1. Indication Selection for Asset Strategy
Selecting indications for a specific molecule is pivotal. AI, in conjunction with RWD, objectively analyzes multiple data sets and foundational models to redefine the art of indication selection. This evidence-based approach identifies novel indications rapidly, providing a holistic foundation for investor and R&D decision-making.
2. Subgroup Discovery for Trial Design
AI and omics-rich RWD facilitate the examination of thousands of genetic and phenotypic attributes, pinpointing combinations likely to influence prognostic or predictive scores. This approach streamlines trial design, reducing duration without compromising success probability, thus expediting treatment availability.
3. Subgroup Discovery and Comparative Efficacy for Portfolio Optimization
AI contributes to a wider portfolio strategy by predicting responses in different patient subgroups. This strategic analysis enhances portfolio optimization, improving net present value and supporting targeted trials. The systematic analysis of available data sources aids in positioning assets optimally in a competitive landscape.
4. End Point Optimization in Clinical Development
In trials with no single established endpoint, AI on patient-level longitudinal datasets identifies attributes closely tracking primary endpoints over time. This approach, incorporating medical imaging and biomarker monitoring, establishes potential novel endpoints and accelerates development timelines.
Overcoming Organizational Challenges
The integration of AI into clinical development poses inevitable organizational challenges. To navigate these challenges effectively:
Embed AI into Clinical Development: R&D leaders can foster a culture change by encouraging analytical methods in decision-making and strengthening development governance models to mandate AI support for key decisions.
Develop Internal Capabilities and Talent: Building internal AI capabilities requires significant investment but ensures independence and agility. Attracting and retaining top data scientists necessitates competitive remuneration and a focus on the industry’s value proposition.
Develop a Targeted Data Strategy: Formulating detailed and iterative data strategies for each disease area ensures effective utilization of diverse data sources, from electronic health records to clinical trials.
Build Scalable Products: AI use cases should be developed as scalable products, facilitating reuse and deployment across the clinical development portfolio. Shared ML development standards and coding frameworks expedite development work.
Conclusion: The Future of Clinical Development
As we stand on the brink of a new era in clinical research, the synergy between AI and clinical development holds immense promise. By embracing the potential of AI and navigating the challenges strategically, the pharmaceutical industry can transcend traditional boundaries, delivering faster access to effective treatments and realizing the true potential of AI-enabled acceleration.
AI/ML Development Services Company, Pitangent, recognizes the transformative power of AI in clinical research and remains committed to contributing to the evolution of drug development through innovative solutions and strategic insights. Embrace the future with us as we break boundaries and shape a new frontier in healthcare.
“Reference: https://postly.link/sHq/”
Frequently Asked Questions (FAQs)
1. How is AI transforming traditional clinical trials in pharmaceutical research?
Answer: AI is revolutionizing clinical trials by enhancing operational excellence and accelerating drug development. It aids in strategic trial design, subgroup discovery, and portfolio optimization, ultimately expediting the delivery of effective treatments to patients.
2. What role does Real-World Data (RWD) play in conjunction with AI in clinical development?
Answer: RWD, including electronic health records, claims data, and diverse patient data sources, forms the foundation for AI applications in clinical development. This data, coupled with AI algorithms, enables more precise trial design, patient subgroup identification, and evidence-based decision-making.
3. How does AI contribute to optimizing endpoints in clinical trials?
Answer: AI analyzes patient-level longitudinal data, incorporating medical imaging and biomarker monitoring. This approach identifies patient attributes closely tracking primary endpoints over time, establishing potential novel endpoints and cutting down development timelines.
4. What challenges does the pharmaceutical industry face in adopting AI for clinical development, and how can these be overcome?
Answer: Challenges include cultural shifts, talent acquisition, data strategy formulation, and the need for scalable AI products. To overcome these challenges, companies can encourage a culture of AI adoption, invest in internal capabilities, develop targeted data strategies, and build scalable AI products for reuse.
5. How is Pitangent, an AI/ML Development Services Company, contributing to the evolution of clinical research?
Answer: Pitangent recognizes the transformative power of AI in clinical research. Through innovative solutions and strategic insights, Pitangent aims to contribute to the evolution of drug development. The company is committed to breaking boundaries and shaping a new frontier in healthcare, embracing the potential of AI-enabled acceleration.