Deciphering Causality in Machine Learning: Crafting Robust Causal Inference Strategies
Posted By - Pony
Posted On - November 24, 2023
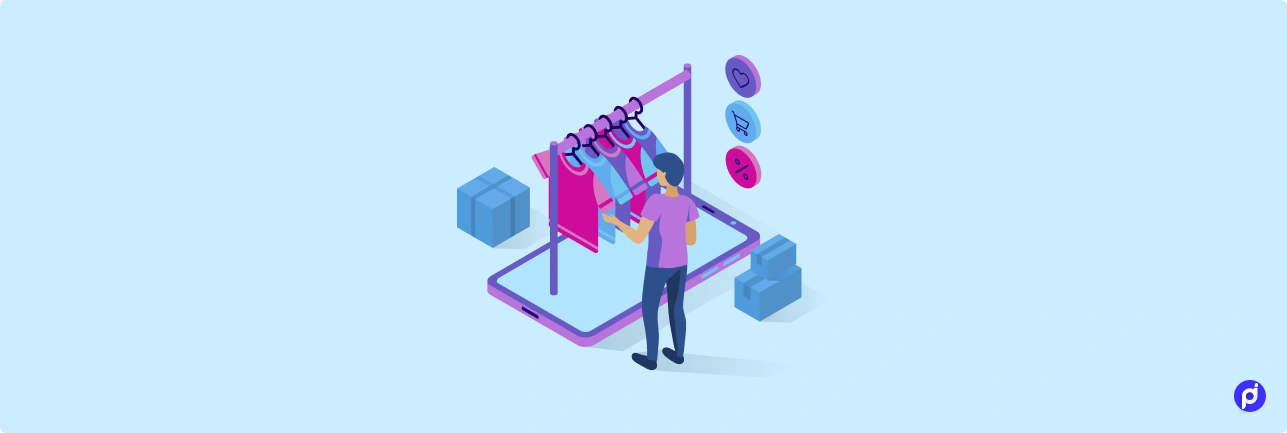
Have you ever found yourself intrigued by the enigma behind your machine learning model’s predictions? What if the training data underwent a transformation? Understanding causality in machine learning is akin to unwrapping the layers of a sophisticated algorithmic puzzle, revealing the concealed connections between variables that shape our predictions.
Cracking the Code: The Essence of Predictive Relationships
Causality in machine learning is a deep dive into cause-and-effect relationships within data, transcending the surface-level associations highlighted by correlation. While correlation establishes links between variables, causality endeavours to unravel the intricacies of why and how one variable influences another. It’s not just about recognizing patterns; it’s about understanding the complex web of connections driving predictions.
Drawing inspiration from François Chollet, we understand that “Causation causes correlation, and correlation, in turn, correlates with causation.” This emphasizes that causation often leads to correlation, indicating a direct influence. However, having a correlation does not guarantee causation; statistical relationships don’t necessarily imply causative ones. This underscores the necessity for meticulous analysis to discern between mere correlation and authentic cause-and-effect relationships in data.
Example Exploration: Navigating Causal Scenarios in Comic Book Sales 🚀
Imagine being the data scientist at Marvel, entrusted with deciphering a sudden surge in comic book sales. Your model excels in accuracy, yet the question lingers: why? Three causal scenarios come to light:
Scenario 1: A Direct Cause 📈 Increased comic book sales directly cause more Disney+ subscriptions. Comics with ads trigger spikes in subscriptions.
Scenario 2: Reversing Cause and Effect 🔄 A rise in Disney+ subscriptions propels comic book sales. Fresh enthusiasts crave more content after discovering characters in Disney+ shows.
Scenario 3: Investigating a Hidden Cause 🕵️ The buzz around Marvel movies on Disney+ stimulates both comic book sales and subscriptions. A concealed cause emerges.
Causal thinking introduces hidden causes, urging models to consider factors beyond apparent correlations.
Real-World Unveiling: Causal Inference in Action 🤔
Causal inference distinguishes itself from conventional statistics by focusing on interventions and actions. Picture this: “Sleeping in shoes causes headaches.” A deeper investigation reveals a confounding variable—a night of drinking—distorting the causal relationship.
In the realm of Causal Machine Learning (Causal ML), an advancing research frontier, the focus lies in enhancing models’ capability to grasp causal relationships in data. Unlike traditional machine learning, which relies on correlations, Causal ML seeks to overcome limitations by navigating the intricate causal web between variables.
Causal ML: Bridging the Chasm Between Correlation and Causation ⚖️
Machine learning models often engage with correlations, exposing patterns while occasionally missing the profound causal understanding beneath. Causal inference emerges to bridge this gap. While machine learning typically identifies patterns (correlations) in data, it doesn’t always illuminate why things occur. Causal Machine Learning (Causal ML) is comparable to upgrading our models to comprehend not just what is transpiring but why. Its aim is to enhance model accuracy and interpretability, holding significant implications for health, economics, policy, and justice.
In conclusion, understanding causality in machine learning transcends the realm of precise predictions. It delves into the ‘why,’ providing insight into the concealed causes influencing our models’ outcomes. As Causal ML evolves, the pursuit to decode the intricate dance of variables persists, promising a future where models not only predict but also comprehend the intricate web of causation.
In the realm of machine learning, the transition from observational data to causal insights is paramount. As a leading ML development Company, Pitangent champions the understanding of causality to construct robust models. Through strategies that prioritize causation over mere correlation, we pave the way for more accurate, interpretable, and impactful machine learning applications.
1. What is Generative AI, and how does it influence the e-commerce shopping experience?
Explore the definition and role of Generative AI in the context of e-commerce, highlighting its impact on enhancing and personalizing the overall shopping experience.
2. Why is social commerce gaining momentum in India, and how are platforms like WhatsApp and Instagram contributing to this trend?
Delve into the factors driving the rise of social commerce, especially among younger demographics, and examine the role of popular social media platforms in shaping online shopping behaviours.
3. How are traditional retailers and e-commerce players adapting to omnichannel retail strategies?
Explore the challenges and opportunities associated with the convergence of online and offline retail channels, emphasizing the importance of providing a consistent shopping experience across various platforms.
4. What are the key technologies reshaping the retail landscape, and how do they contribute to a seamless shopping experience?
Examine the role of new and emerging technologies, including Generative AI, machine learning, and big data analytics, in reshaping user experiences in online shopping and optimizing supply chain processes.
5. What are the growth prospects for the Indian retail and e-commerce sectors in 2024, and what factors contribute to this positive outlook?
Provide insights into the optimistic forecast for the industry in 2024, citing the continued adoption of technology, increasing consumer comfort with digital platforms, and the impact of e-commerce growth in smaller cities and towns.