Data Analytics Solutions: Mitigating Credit Risk and Ensuring Compliance with Data Science and Predictive Modeling
Posted By - Pony
Posted On - August 1, 2023
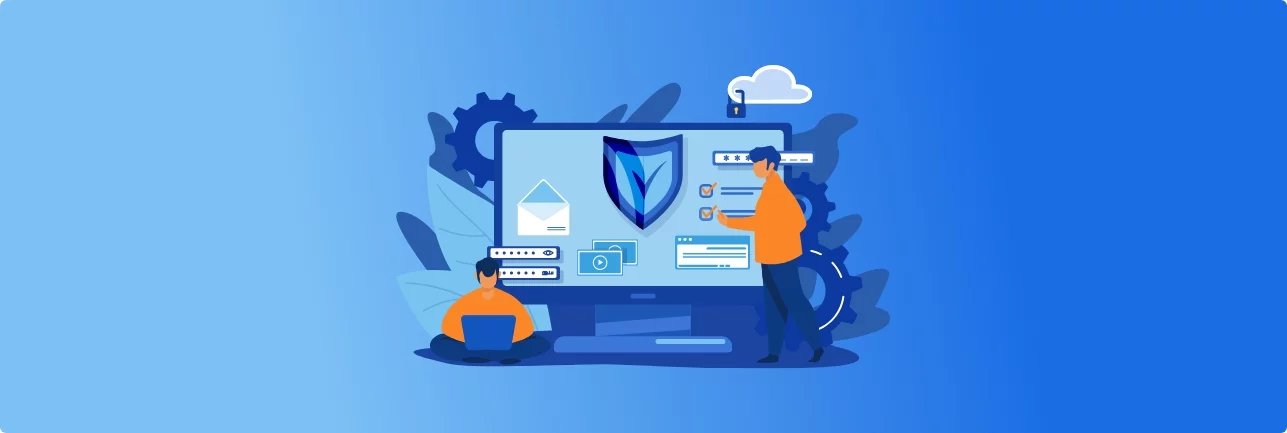
Amidst the rapidly evolving financial services industry, businesses are facing pressing concerns in ensuring robust credit risk assessment and fraud detection capabilities. These aspects have become vital for safeguarding their operations and ensuring a secure environment. The need to accurately evaluate creditworthiness and identify potential fraudulent activities has never been more pressing. To address these challenges, financial institutions are turning to advanced data analytics solutions in Queensland. In this blog, we will explore how leveraging data science and predictive modelling can help mitigate credit risk and ensure compliance, ushering in a new era of secure and responsible lending practices.
Let’s start!
How leveraging data science and predictive modelling can help mitigate credit risk and ensure compliance?
Enhanced Credit Risk Assessment through Data Analytics Solutions
Traditional credit risk assessment methods often rely on historical data and subjective evaluations, making them susceptible to human errors and biases. Data analytics solutions in Queensland, on the other hand, offer a data-driven approach that combines historical data with real-time information to generate more accurate credit risk assessments.
By conducting an analysis of vast amounts of customer data, including transaction history, payment behaviour, and credit utilization patterns, predictive modelling algorithms can identify potential default risks and delinquencies. These insights empower financial institutions to make informed lending decisions and offer personalized credit terms that align with borrowers’ risk profiles.
Real-time Fraud Detection and Prevention
Fraudulent activities can inflict significant financial losses on financial institutions and their customers. Data analytics solutions in Queensland play a crucial role in combatting fraud by continuously monitoring transactions and identifying suspicious patterns in real-time.
Using machine learning algorithms, these solutions can detect unusual behaviour, such as unauthorized transactions or identity theft, and trigger alerts for immediate action. As a result, financial institutions can respond swiftly to potential fraud attempts, safeguarding their assets and protecting their customers from financial harm.
Unearthing Hidden Insights with Predictive Modeling
Predictive modelling goes beyond traditional credit scoring by delving into a borrower’s overall financial behaviour. By incorporating non-traditional data sources such as social media activity and online purchasing behaviour, data analytics solutions can gain deeper insights into an applicant’s creditworthiness.
This approach is particularly beneficial for individuals with limited credit histories or those seeking alternative financing options. Financial institutions can leverage predictive modelling to assess risk more accurately and extend credit to underserved populations responsibly.
Ensuring Compliance with Regulatory Requirements
The financial services industry operates within a web of stringent regulations to protect both consumers and financial institutions. Compliance with these regulations is paramount to avoid legal consequences and maintain a trustworthy reputation.
Data analytics solutions in Queensland can assist financial institutions in adhering to regulatory guidelines by automating compliance checks and audits. These solutions can identify potential violations, ensure adherence to anti-money laundering (AML) and know-your-customer (KYC) regulations, and streamline the reporting process.
Proactive Portfolio Management and Risk Mitigation
Data analytics solutions offer continuous monitoring of a financial institution’s portfolio, allowing proactive identification of potential credit risks. By regularly assessing the creditworthiness of borrowers, financial institutions can take timely measures to mitigate risk and prevent defaults.
Additionally, predictive modelling can simulate various economic scenarios to evaluate their impact on the portfolio’s overall risk exposure. Armed with this information, financial institutions can make strategic decisions to optimize their risk-return profiles and maximize profitability.
Conclusion
The challenges of credit risk assessment and fraud detection in the financial services industry call for innovative solutions that can keep pace with rapidly evolving threats. Data analytics solutions in Queensland, Australia offer the power of data science and predictive modelling to tackle these challenges head-on. By leveraging the insights derived from vast amounts of customer data, financial institutions can make informed credit decisions, identify potential fraud in real-time, and ensure compliance with regulatory requirements. Embracing data analytics solutions not only mitigates credit risk but also strengthens the foundation of responsible lending practices, driving the industry toward a more secure and prosperous future.