The Dos and Don’ts of Implementing Data Science Services
Posted By - Pony
Posted On - October 16, 2023
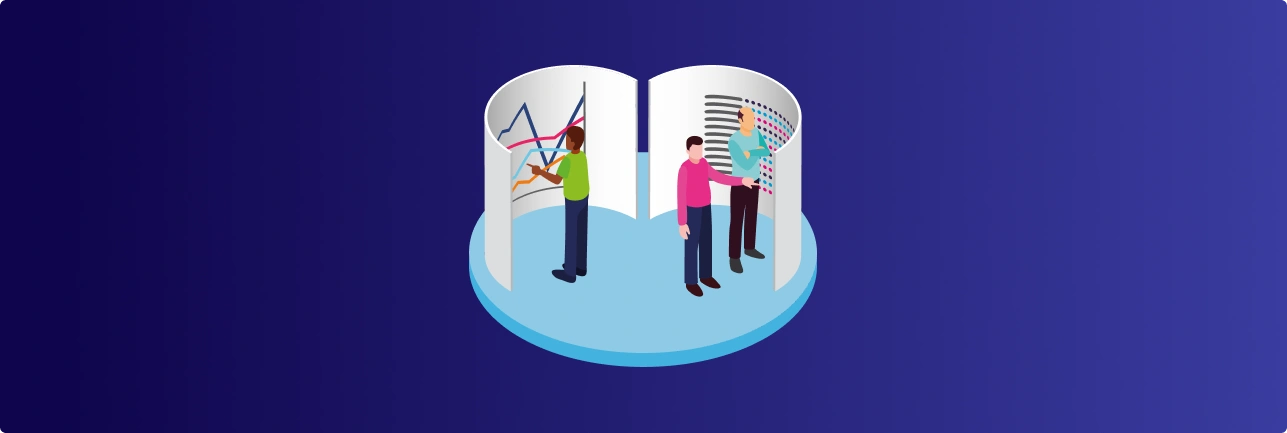
Data Science is a hot topic in business. It is widely used in various industries. To gain competition many businesses are embracing it without any hesitation. But what haunts them is how to implement it. However, the successful implementation of these services requires careful consideration and a clear strategy If you are facing the same issue then follow the description. Here we bring forward some key do’s and don’ts of implementing Data Science Services to ensure your business maximizes its potential.
Here are the Do’s
Dos
Understand Your Business Goals
Before diving into Data Science Services, it’s crucial to align the implementation with your business goals. Ask yourself what specific problems or opportunities you hope to address with these services. Are you seeking to improve customer retention, optimize supply chain operations, or enhance product recommendations? Defining your objectives will guide the entire process.
Data Quality Is Key
High-quality data is the lifeblood of any successful data science project. Ensure that your data is clean, accurate, and well-structured. Data preparation and cleaning are often the most time-consuming aspects of any project, so investing in this phase will save you time and resources down the road.
Choose the Right Data Science Services Provider:
If you lack in-house expertise, consider partnering with a Data Science Consulting firm. When selecting a service provider, look for a company with a proven track record in your industry. They should have a team of experienced data scientists and machine learning engineers who understand the unique challenges and opportunities within your sector.
Start Small, Scale Gradually
It’s tempting to launch a comprehensive data science project, but starting small and scaling as you go is often a safer approach. Begin with a well-defined pilot project to test the waters and understand the potential ROI. As you gain confidence and insight, you can expand your initiatives.
Continuous Learning and Adaptation
Data science is a rapidly evolving field. To stay competitive, encourage your team to continuously learn and adapt. Attend industry conferences, invest in training, and promote a culture of innovation within your organization.
Don’ts
Ignoring Data Privacy and Security
In the rush to harness the power of data, do not overlook the importance of data privacy and security. Failing to protect sensitive customer information can lead to legal issues and reputational damage. Ensure your data handling practices comply with industry regulations and best practices.
Overlooking Interpretability
Many data science models, especially in machine learning, can be complex and difficult to interpret. Focusing solely on predictive accuracy without understanding the model’s decision-making process can lead to misguided actions and unintended consequences.
Neglecting Stakeholder Involvement
Data science projects should not be siloed within your IT department or with external consultants. Involve stakeholders from various parts of your organization, including marketing, sales, and operations. Their input is invaluable in ensuring the data science solution aligns with the business’s overall strategy.
Rushing the Process
Implementing data science is not a race. Rushing through the process can lead to subpar results and wasted resources. Take the time to plan, execute, and refine your projects for the best outcomes.
Lacking a Clear Measurement and Monitoring Plan
Once your Data Science Services are up and running, it’s essential to monitor their performance continually. Establish key performance indicators (KPIs) and regularly assess whether the services are meeting your business goals. Without proper measurement and monitoring, you won’t know if your investment is paying off.
Let’s finish off
The successful implementation of Data Science Services, whether for AI/ML development or other applications, requires careful planning and execution. By adhering to the dos and don’ts outlined in this blog, your business can harness the power of data science to gain a competitive advantage, drive innovation, and make data-driven decisions that will propel you ahead in your industry.
Here are the 5 FAQ’s
Q1: What is the first step in implementing Data Science Services for my business?
A1: The first step is to understand your business goals. Define the specific problems or opportunities you want to address with data science. Whether it’s improving customer retention, optimizing supply chain operations, or enhancing product recommendations, aligning your objectives is crucial to guide the entire process.
Q2: Why is data quality important in data science projects?
A2: Data quality is crucial because it forms the foundation of a successful data science project. High-quality data, which is clean, accurate, and well-structured, ensures that the insights and models developed are reliable and meaningful. Investing in data preparation and cleaning at the outset saves time and resources in the long run.
Q3: Is it necessary to partner with a Data Science Services provider if we lack in-house expertise?
A3: If you lack in-house expertise, partnering with a Data Science Consulting firm can be a good strategy. When choosing a provider, look for one with a proven track record in your industry, experienced data scientists, and machine learning engineers who understand your sector’s unique challenges and opportunities.
Q4: What’s the importance of involving stakeholders in data science projects?
A4: Involving stakeholders from various parts of your organization, such as marketing, sales, and operations, is essential. Their input ensures that the data science solution aligns with the overall business strategy, increasing the chances of success and adoption.
Q5: Why should businesses start small and scale gradually with data science initiatives?
A5: Starting small and scaling gradually is often a safer approach because it allows you to test the waters with a well-defined pilot project. This helps you understand the potential return on investment (ROI) and minimizes risks. As you gain confidence and insight, you can expand your data science initiatives in a more informed manner.