Decoding Efficiency: Harnessing Data Analytics in Semiconductor Manufacturing
Posted By - Pony
Posted On - November 24, 2023
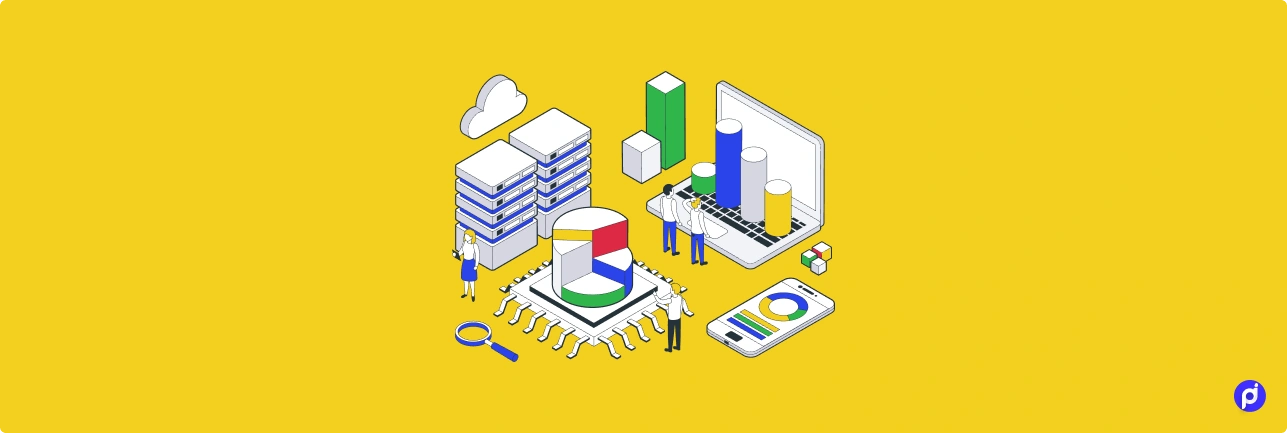
In the dynamic landscape of the semiconductor industry, the demand for flawless chips is more critical than ever, especially as these tiny marvels find their way into applications with potential life-and-death implications, such as automotive systems. With defects measured in parts per billion, the need to completely eliminate flaws from the manufacturing chain has become urgent, highlighting the delicate balance between quality and quantity.
The Urgency of Defect Elimination
Semiconductors have become the backbone of various industries, controlling intricate systems in automobiles and beyond. The smallest defect can have severe consequences, necessitating a paradigm shift towards a defect-free production environment. As the industry experiences unprecedented growth, the challenge lies in meeting the escalating demand for chips without compromising quality.
The Role of Data Analytics in Quality Enhancement
Harnessing the power of data analytics has emerged as a game-changer in semiconductor manufacturing. While data collection is a routine part of the process, the key challenge lies in transforming this data into actionable insights. The entire semiconductor lifecycle, from design to production, can benefit from data analytics to enhance performance, decrease bring-up time, and boost overall quality and productivity.
The Accenture Insight
According to Accenture,
“the application of analytics in semiconductor manufacturing operations has the potential to cut machine downtime in half. Furthermore, the integration of analytics and machine learning technology could increase production yield by a substantial 5 to 15 percent. This emphasizes the substantial benefits that lie in the effective use of data analytics throughout the manufacturing chain.”
Key Benefits of Data Analytics in Semiconductor Manufacturing
- Identification of Improvement Metrics: Data analytics allows the identification of production metrics with potential for enhancement, paving the way for targeted improvements.
- Outlier Detection: By flagging data outliers related to production issues, analytics acts as a proactive measure to address potential defects.
- Root-Cause Analysis: Data analytics facilitates comprehensive root-cause analysis across the entire manufacturing chain, addressing issues at their source.
- Consolidation of Analytics: The consolidation of analytics across the manufacturing chain ensures a holistic approach, allowing for a more comprehensive view of potential challenges.
- Speed-to-Market Acceleration: The application of data analytics accelerates speed-to-market, enabling more efficient customization of products to meet diverse demands.
Leveraging AI for Enhanced Analytics
The integration of AI and machine learning technologies enhances the value derived from semiconductor data. Leading manufacturers are leveraging predictive AI to anticipate errors and equipment breakdowns, resulting in improved quality and yield. Despite the staggering productivity improvement potential, only 26% of manufacturers currently utilize AI-powered analytics, according to Gigaphoton.
Challenges and Opportunities
The broader adoption of data analytics faces challenges in hardware, software, and data management. Hardware variations and software fragmentation pose hurdles, while inefficient data management affects insight generation. A unified approach, represented by Fabscape’s maturity model, addresses these challenges through a structured progression from data initiation to AI-driven insights.
Fostering a Future of Zero-Defect Production
As standards and models, such as those proposed by Fabscape, gain traction, the semiconductor industry inches closer to a future of zero-defect production. The strategic application of data analytics across the manufacturing chain, supported by AI technologies, promises not just efficiency but a fundamental shift towards a more productive and reliable semiconductor ecosystem.
In conclusion, the journey towards decoding efficiency in semiconductor manufacturing involves more than just data collection—it requires a meticulous application of analytics and AI technologies. By addressing challenges in hardware, software, and data management, manufacturers can pave the way for a future where defects are minimized, if not eliminated entirely. The evolution towards zero-defect production is not just a goal; it’s a commitment to delivering the highest quality semiconductor products in an era where their applications are more critical than ever.
Data Analytics Service Providers play a pivotal role in navigating these challenges, offering tailored solutions for efficient data management and analytics implementation.
” For reference: https://postly.link/9pS/ “
- What is the significance of data analytics in semiconductor manufacturing?
- Answer: Data analytics plays a crucial role in semiconductor manufacturing by transforming vast amounts of production data into actionable insights. These insights contribute to improving performance, reducing bring-up time, and enhancing overall quality and productivity throughout the manufacturing chain.
- How can data analytics address the urgent need to eliminate defects in semiconductor production?
- Answer: Data analytics enables the identification of production metrics with improvement potential, facilitates outlier detection related to production issues, and allows for comprehensive root-cause analysis. By consolidating analytics across the manufacturing chain, it offers a holistic approach, accelerating the industry’s journey towards zero-defect production.
- What benefits can semiconductor manufacturers expect from leveraging AI in analytics?
- Answer: The integration of AI and machine learning technologies enhances the value derived from semiconductor data. Manufacturers can use predictive AI to anticipate errors and equipment breakdowns, resulting in improved quality and increased production yield. Despite its potential, the adoption of AI-powered analytics is currently utilized by only 26% of manufacturers, according to a report from Gigaphoton.
- What challenges do semiconductor manufacturers face in adopting data analytics, and how can they be addressed?
- Answer: Manufacturers encounter challenges in hardware variations, software fragmentation, and inefficient data management. The lack of a standardized analytics platform and varying capabilities of hardware and software components pose hurdles. To overcome these challenges, Fabscape’s maturity model suggests a phased approach from data initiation to AI-driven insights, addressing gaps in hardware, software, and data management.
- How can Data Analytics Service Providers contribute to the advancement of analytics in semiconductor manufacturing?
- Answer: Data Analytics Service Providers are instrumental in navigating challenges related to data management and analytics implementation. Their tailored solutions can help semiconductor manufacturers efficiently collect, manage, and analyze data, ensuring a smooth transition towards a future of zero-defect production.